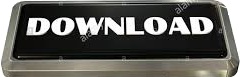

Where the one-hot encoding happens, is within the _getitem_ method. The constructor accepts predictors, labels, a fitted OneHotEncoder, batch_size, the number of classes, a maximum sequence length and a boolean to shuffle.ĭef _init_(self, predictors, labels, enc, batch_size=32, n_classes=25, max_seq_len=25, shuffle=True): The class DataGenerator inherits from Sequence, because it’s a very memory-efficient and structured way of generating batches. As a matter of fact, it’s not even that hard. I wrote a DataGenerator class that properly encoded the labels. However, this week, I solved the problem.

Tensorflow custom data generator generator#
Resource exhausted: OOM when allocating tensor with shape and type floatĪlthough it as clear to me I should use a generator (like the ImageDataGenerator), my experience with writing custom TensorFlow code was limited. Tensorflow/core/framework/op_:1502] OP_REQUIRES failed at one_hot_op.cc:97 : Tensorflow/core/framework/:107]Īllocation of 18970130000 exceeds 10% of system memory. These are the two errors I kept running into: After tokenizing the predictors and one-hot encoding the labels, the data set became massive, and it couldn’t even be stored in memory. I had everything figured out, but tokenizing the text and one-hot encoding the many labels was an issue. Basically, it’s a model that predicts what the next word should be in a sentence. Some months ago, I tried training a text generator on a huge corpus of text with an LSTM model.
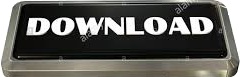